I’ve been in my new post in the Geography department at King’s College London for nearly nine months now and — together with another new-ish colleague — have been asked to design a programme to teach quantitative research methods to students who often seem to think that their interests are solely qualitative. On the positive side, it’s exciting to be somewhere that sees quantitative methods as being (again) part of the future and not just a resurrection of a failed positivist tradition. On the negative side, it’s daunting to realise just how far some students are from being comfortable with computers, data, or statistics.
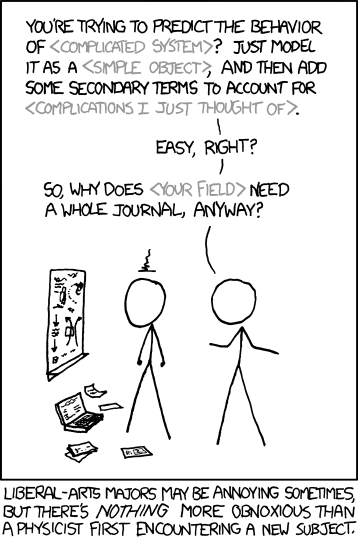
In this post I want to talk generally about the challenges facing geography students. In the next post(s) I will discuss specific issues in teaching quantitative methods to non-quantitative students, and some of the ideas that I’m currently working on to try to address them.
The Computer Skills Challenge
One particularly thorny problem facing anyone trying to get students started with quantitative methods has been the reform of computing instruction. A report by the Royal Society titled ‘Shut Down or Restart‘ highlights the challenges students have in trying to acquire useful computing skills at the secondary level. The situation is apparently so dire than only 1 in 8 ‘elite’ universities even lists the computing A-level as a requirement for entry to a Computer Science program.
As a graduate of a liberal arts programme where I learned how to programme as part of an English class I’m not actually certain that entry requirements are in the best interests of students or universities, but in the more specialised English system the absence of such a requirement is a clear indication of a problem finding the right intake. The underlying issue is that existing classes are not teaching students how to do much with a computer beyond some light work in MS Word and (if you’re lucky) Excel. To be fair, government seems to have finally recognised that using computers is not the same as understanding them.
Almost as important for the long run is the arrival Raspberry Pi, which offers a way for computers to be made simple and cheap enough that they can be breakable. That might sound a little strange, but I think one of the things that is crucial to learning computing is play. And you can’t play with a computer that is locked to a desk, net boots, is completely locked down by access controls, and whose use is tightly supervised by a teacher who may also have comparatively very little comfort with the devices themselves. Only when children can break stuff without fear of consequence can they experiment in ways that will excite them. Set them loose and I hope that we might see the gap between the number of computationally capable students that we need and the number that we actually have begin to close.
To me, this gap matters because there is no question that computational tools will underpin many of the inventions and innovations of the next fifty years. And the crucial difference from the previous fifty years is that these methods will not be confined to ‘historically’ computer-intensive fields such as bioinformatics, computer science, or particle physics. The direct consequence of our love for all things digital is that — thanks to the data spewed out as a byproduct of activity on mobile phone networks and social networks, as well as processes such as the digitisation of old manuscripts — computational methods can now be brought to bear on topics that were largely unaffected by the first wave of computing.
Geography — especially human geography — is one such discipline; computational methods are of increasing importance and, fortunately, the ESRC recognizes this.
Space, Place & Computation
Students and researchers in Geography are going to encounter computational methods — if they haven’t already — more and more frequently in their work. It will, of course, partly be the result of a faddish preference for something shiny and new. But to dismiss the current interest in ‘big data’ as ‘Quantitative Geography 2.0’ is to seriously underestimate the extent to which the ground has shifted thanks to the radically expanded power of computers & algorithms. In spite of my post on ‘Big Data’s Little Secrets’, the fact remains that the extent to which everything that we do now leaves a digital trail across time and space means a sea change in the field.
If you’re in the mood for some light entertainment (and good editing, though nothing can fix the fact that it had been too long since my last haircut) you can hear me try to say all of this in one take here:
Let me illustrate this using an example with which I am somewhat familiar: travel. Until very, very recently, if you wanted to know how people were getting from A to B you had to ask them directly. But travel demand surveys are rather difficult to administer: do you try to bother people at home? Do you try to bother them on their way to work? Or do you only update your data every few years when the latest Census or mid-year estimates come out and allow you to tie households to offices (if they have one).
Compare that situation to what is possible with Oyster data: with Oyster you’d get roughly 80-85% of all public transit users in London. If they are rail users (i.e. Tube, Overground & [to a lesser extent] National Rail) then you already know (roughly) where they entered the Oyster charging zone and (roughly) where they exited it… If they are bus or tram users then the locational data is a bit weaker, but not for long since the Oyster readers have now been synched up with the GPS units on buses. Interestingly, it turns out that the vast majority of people will begin their next transit journey with a tap-in that is quite close to the point where their previous journey ended. In other words, you can guess where someone exited the bus based on where they next boarded some other form of public transit[1].
Of course, I am not arguing that Oyster data tells us why people are travelling, but if we take this as a starting point then the travel survey of the future could look utterly different from what we do now. And more importantly, the quantitative results are comprehensive enough that they can and should inform the qualitative research (including who is not being picked up in the Oyster data).
The same issue applies to all sorts of other geographical research: do you really need people to keep a detailed daily diary when you could log their movements automatically on their phone in far more detail and ask them from time to time what they are doing? Something like Mappiness, perhaps? Or for the more physical or wildlife-oriented types: coupling high-resolution cameras to a cheap, UAV or automated drone could give you the ability to capture and process all sorts of remote environmental data in a matter of minutes, and to do so without disturbing the flora or fauna. This survey work would previously have taken days or months of work on the ground or at sea and it can now be done in a fraction of the time, or at a fraction of the cost.
Quantitative Teaching in Context
I hope I’ve made a convincing case in this (and other) posts for the need for such teaching at the undergraduate level in British universities. But these developments leave a forward-looking geography department in a quandary: for the foreseeable future many of their first years will not have taken a maths, sciences, or computing A-level and so will have had little or nothing to do with the natural sciences since GCSE. The easy way out would be to dumb down the quantitative material so that students don’t feel too threatened or challenged and, with luck, pick up a couple of bits of knowledge along the way. What we really need though are ways to ‘level-up’ all students: we need to find ways of teaching quantitative material that engage all types of learners and empower students with the realisation that they can do this.
Ways of doing that will be the focus of my next post(s).
Summary
There are many promising signs on the horizon that a disciplinary pendulum that has swung a long way in one direction is slowly starting to swing back. What we need to avoid, however, is the pendulum swinging so far back that the gains from cultural geography are largely lost or neglected in the same way that those from quantitative geography have been. It might seem unlikely now, but in the pursuit of academic fashion, I wouldn’t be surprised if this were a risk in a few years time. For me, the best possible outcome of this resurgence would be to see students employing qualitative research methods as their principal source of data, but nonetheless applying quantitative approaches to: test their results for significance; select their case studies; identify bias in their interviewees…
What do you think? Can these two traditions arrive at a place of mutual respect, and is making quantitative methods more accessible one of the ways to achieve this?
[1] This makes sense because if you are interchanging with a rail system then you’ll likely board or exit the bus near the station, and if your next journey is on a bus then it’s likely to either be an interchange or the beginning of your return journey!